Overview: Purpose and Ideation
The purpose and ideation phase sets the direction and foundation for your AI solution, aligning it to the needs of those most impacted by its outputs. Considering equity throughout the ideating activities (defining the problem, setting goals, and aligning use cases) creates the conditions for an AI system that prioritizes fairness, inclusivity, and transparency.
As chief information officers increasingly experiment with AI projects, nearly half of these initiatives fail to successfully transition from pilot phase to full-scale implementation.1 One source of these failed deployments is the misalignment of business needs with the AI use cases, and research identified it as one of the most significant barriers to AI adoption.1 2 Defining clear, equity-aligned use cases begins with an in-depth exploration of the problem and how the objectives for the AI solution fit within the broader context of organizational goals.
Problem Definition
AI solutions are complex and often costly to develop and implement. Engaging in problem definition before developing an AI solution ensures alignment with stakeholders' needs, prevents solution bias, and enables the creation of effective and meaningful solutions that address the root causes of the problem while keeping costs within budget. From an equity standpoint, a well-defined problem statement that recognizes the existing strengths and identifies opportunities to build on them helps mitigate the risk of biases and misuse of AI outputs while centering the experience and needs of those most impacted by the solution. By prioritizing equity in problem definition, developers can strive for fair and inclusive AI solutions that contribute positively to diverse user experiences and societal well-being.
Asset-based Approach
Taking an asset-based approach in crafting a problem statement fosters a more empowering and inclusive framework for problem-solving. The approach recognizes and builds on existing capabilities by focusing on assets, strengths, and resources within user groups rather than solely on deficits or challenges. This approach encourages collaboration and innovation. The problem statement should explore how AI can serve as a tool to enhance and amplify the strengths and resources present.
Consider this problem statement:
"Our university faces a significant challenge with student engagement and retention rates, particularly among underrepresented minority groups. How might implementing an AI-driven surveillance system to monitor student attendance and behavior patterns support faculty and administrators when students exhibit signs of disengagement?”
This problem statement takes a deficit-based approach and frames engagement and retention as a student challenge. As a result, the proposed AI solution aims to mitigate the problem of low engagement and retention rates by monitoring and identifying students at risk. It does not recognize or leverage the existing capabilities and resources present within the student body. Instead, it relies on surveillance and intervention measures, which may not foster an empowering or inclusive environment for problem-solving.
Consider this problem statement instead:
“Our student body possesses a wide range of talents, skills, and diverse backgrounds that, when recognized, can foster dynamic, collaborative learning environments. Traditional curriculum delivery methods do not provide opportunities for students to understand their individual strengths and collaborate with peers, leading to disengagement. How can we utilize AI-driven personalized learning platforms to adapt to individual learning styles and increase opportunities for collaboration?"
This problem statement adopts an asset-based approach, recognizing the diverse strengths and resources within the student community. It shifts the “engagement issue” from a deficit approach to one that identifies opportunities for a solution to amplify these assets by providing personalized learning experiences that cater to individual differences, cultural backgrounds, and skill levels.
Addressing the Root of the Problem
Problem statements should address root causes to focus efforts on resolving underlying issues to prevent wasted resources and avoid exacerbating existing equity gaps over time. Failure to address the root cause can lead to ineffective solutions, increased costs, and diminished trust in the organization's ability to address challenges.
Consider this problem statement:
"Low retention rates among first-generation college students indicate a need to increase access to 24/7 general student support. How might we use an AI chatbot system to provide quick access to common student questions related to registration, financial aid, and academic resources?"
This problem statement does not address the root cause of the low retention rates. Instead, it suggests addressing a symptom, low retention rates, with a solution, an AI chatbot for faster general support services, that may not directly tackle the underlying issues faced by first-generation students, such as lack of tailored support and feelings of isolation.
Consider this problem statement instead:
"Low retention rates among first-generation college students at our university stem from a lack of support services tailored to their unique needs, leading to feelings of isolation and academic unpreparedness. How can we leverage AI to implement a mentorship program that provides personalized academic and social support to first-generation students?"
This problem statement addresses the root cause of low retention by identifying the lack of personalized support for first-generation students that may lead to feelings of isolation. From here, the organization can set goals and objectives for an AI solution addressing these root causes and understanding the problem.
Reference this resource we created, Problem Definitions Guiding Questions to guide your discussion at this phase. 3
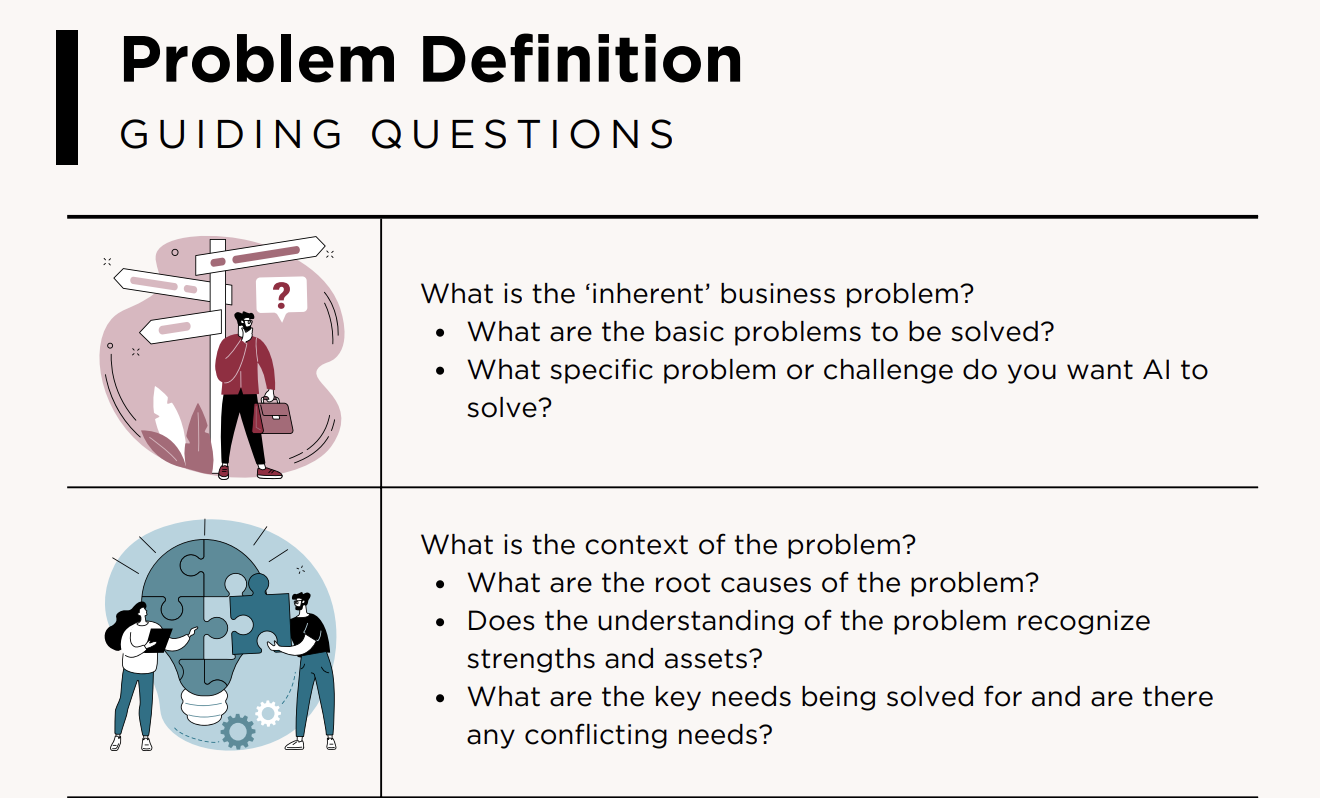
Goal Setting and Approach
The goal-setting process lays the groundwork for success, contextualizing the goals for the AI solution within the broader organizational goals to help clarify distinct use cases for the solution. Goal-setting requires strategic planning, leadership support, stakeholder input, and specialized expertise. Despite the time investment required, a thoughtful and inclusive goal-setting process is important to developing relevant and sustainable solutions that meet the needs of all stakeholders involved.
In aligning goal-setting with broader institutional objectives, goals should be mapped to AI solution objectives at all levels, catering to various stakeholder groups. This activity necessitates a strong partnership between institutional leaders and solution providers, fostering collaboration across departments and a defined strategy to manage relationships and potential conflicts. Framing AI goals as hypotheses or well-posed questions helps focus on specific benefits for the various user groups.4
Hypothesis-framed example: Instead of a general goal like "Implement AI for student retention," framing it with an equity focus could be: "Developing an AI-driven student support system that identifies students in need of support from marginalized backgrounds early in their academic journey and provides tailored interventions will narrow the achievement gap by 25% and improve retention rates for marginalized student populations." This hypothesis emphasizes the importance of addressing equity gaps in higher education and sets specific targets for improving outcomes for marginalized students.
For more information on stakeholder mapping, visit the Stakeholder Engagement section of this guide.
.png)
Clear goals drive the institution's strategy and theory of change, leading to the identification of relevant use cases. This approach minimizes the risk of misaligned use cases that plague many AI solutions1 by ensuring they stem from the overarching goals of the institution. Beginning with broader institutional goals to delineate specific objectives for the AI solution and potential use cases, the focus shifts from the technology to a more student-centric approach.
Reference this resource we created, Goal Definition Example to guide your discussion at this phase.
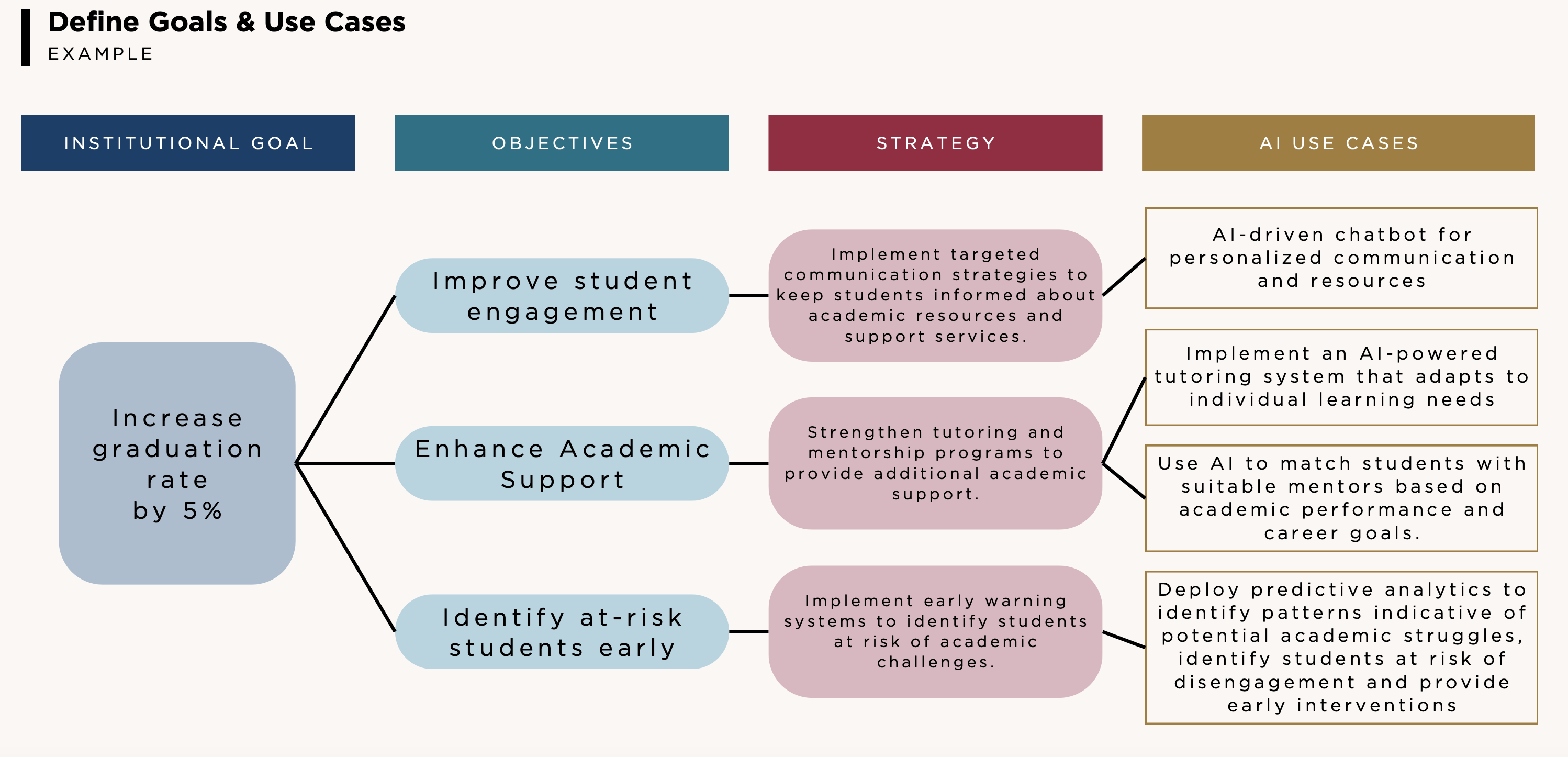
Taking a targeted universalist approach to goal definition supports the development of equitable solutions. Clearly defining goals for these unique subpopulations transforms the solution from a neutral or universal benefit for all students to an equitable solution that acknowledges various student groups' unique needs and challenges.
Use Case Definition
The use cases defined during the purpose and ideation phase of development will guide decision-making throughout the development and implementation phases. Having a clear and shared understanding of the intended use for the AI solution, how it aligns with institutional goals, and potential ways it could be misused will support the development team in mitigating bias throughout the process. Beyond development, clearly defined use cases enable transparent stakeholder engagement, enhance the explainability of the solution, and allow for continuous evaluation of the project. Defining use cases involves exploring all possible ways that AI can address the goals and strategies defined during goal-setting while being mindful of preventing outputs from causing unintended harm.
Imagine, for example, a higher education institution developing an AI solution to streamline and optimize financial aid decisions to benefit the institution and students. The intended use case is to analyze factors, such as academic performance, family income, and other relevant data, to allocate financial aid resources fairly to students in need. However, due to a lack of clear guidelines and oversight, the AI solution is applied to a different use case. Instead of focusing on fair and equitable distribution of financial aid, the institution leverages the AI system to identify applicants more likely to graduate and contribute financially to the institution in the long run. This misaligned use case is driven by a desire to increase graduation rates and maximize revenue rather than prioritizing the financial needs of students.
In this scenario, the AI system, originally designed to help students experiencing poverty, failed to recognize the systemic factors that contributed to the previous academic performance of students experiencing poverty. Instead of providing the intended benefits, the system disadvantages these students. The system might inadvertently favor applicants from wealthier backgrounds, even if they don't necessarily require financial aid, in an attempt to secure future financial contributions to the institution. This misalignment could lead to a biased and unfair distribution of financial aid, hindering the academic pursuits of deserving but financially disadvantaged students.
The importance of clearly defined use cases becomes evident in this example, as it highlights the potential ethical and social consequences of deploying AI solutions without a robust framework. Comprehensive guidelines and effective oversight help ensure the ethical application of AI technologies and their alignment with intended purposes, safeguarding against unintended harm and fostering fairness in decision-making processes.
Process for defining use cases for AI
- Expectations: When defining use cases for AI, it's crucial to set clear and realistic expectations by outlining specific objectives, desired outcomes, and measurable success criteria to ensure alignment with stakeholders' needs and avoid overpromising capabilities.
- Primary Function: An AI solution has the potential to impact stakeholders at every level of the institution; therefore, one should consider whether the tools are student-focused, faculty and staff-focused, or institution-focused. Identifying the focus stakeholders is the foundation of the stakeholder mapping process.
- Use case categories: There are endless possibilities for applying an emerging technology, so it may be helpful to focus on a particular overarching category of AI use cases: process automation, cognitive engagement, and cognitive insight.5
- Types of AI: Beyond the general categories of AI uses, exploring the different types of AI can open up new opportunities as you consider potential use cases to solve your core business problems and achieve your goals. The five types of AI considered here are Text AI, Visual AI, Interactive AI, Analytical AI, and Functional AI.
Different types of AI excel in specific tasks, with machine learning and deep learning being particularly effective in data analysis and forecast(predictive) modeling. However, these models are often considered "black boxes," where it is challenging to understand the decision-making process, making them less trustworthy and transparent to users. Additionally, their reliance on large amounts of data raises concerns about data protection and privacy, especially in sectors with increasing privacy regulations internationally. Those looking to delve into AI must weigh the promise and potential benefits of these technologies against the limitations and potential risks.5
For more information on assessing potential risks, visit the Risk and Fairness section of this guide.
Reference this resource we created, Use Case Definition Worksheet, to guide your discussion at this phase.
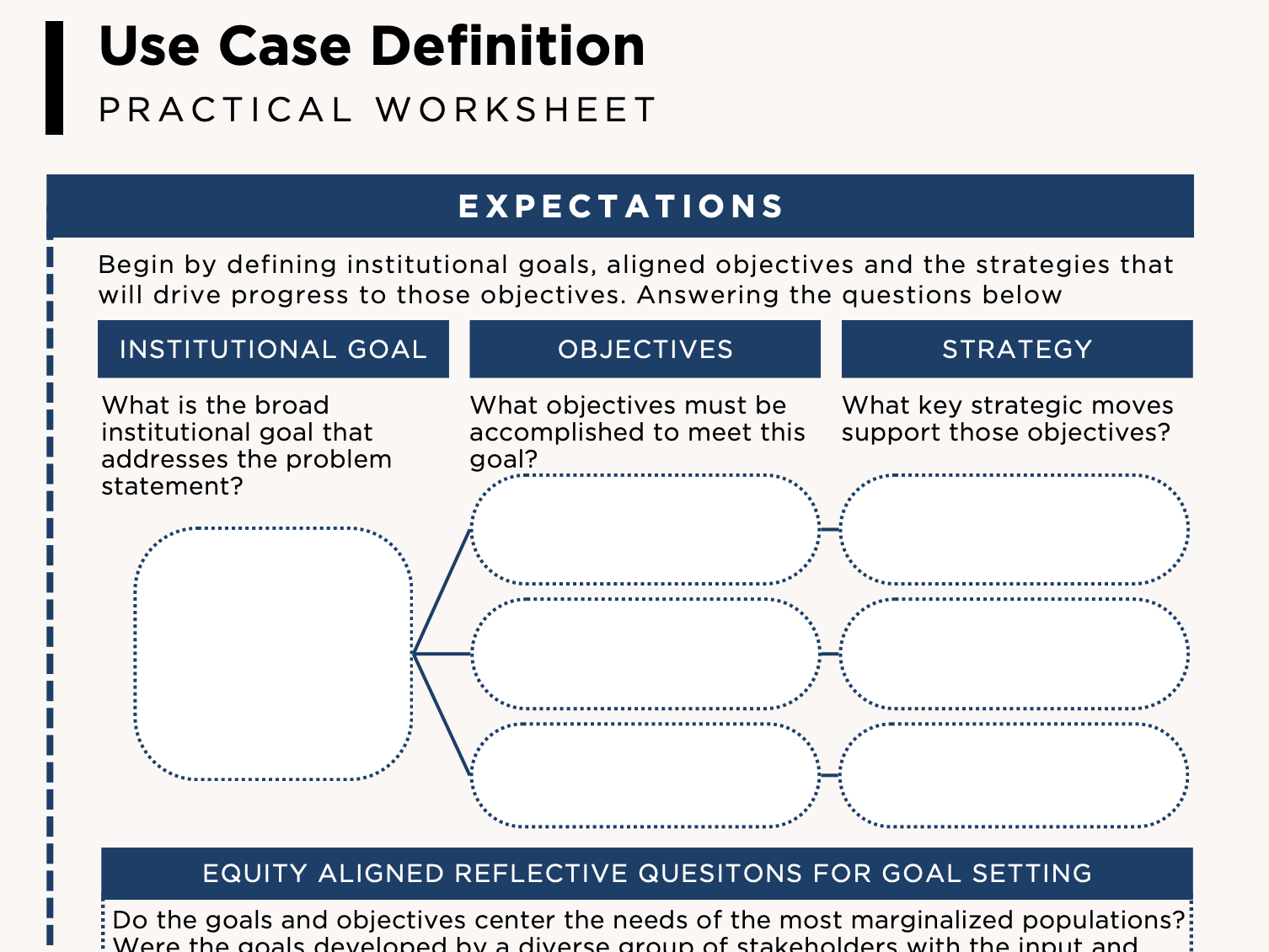
AI as a Solution
AI has the potential to solve complex problems; however, it may not be the best solution in all situations. Assessing the suitability of AI for a given problem from an equity perspective is fundamental for responsible AI development. It helps align technological advancements to create fair, inclusive, and socially beneficial solutions. This suitability assessment includes several pre-development considerations to determine if AI as a solution is viable, appropriate, advantageous, and equitable when considering the associated risks of unintended harm.
Reference this resource we created, Considerations for AI as a Solution, to guide your discussion at this phase.
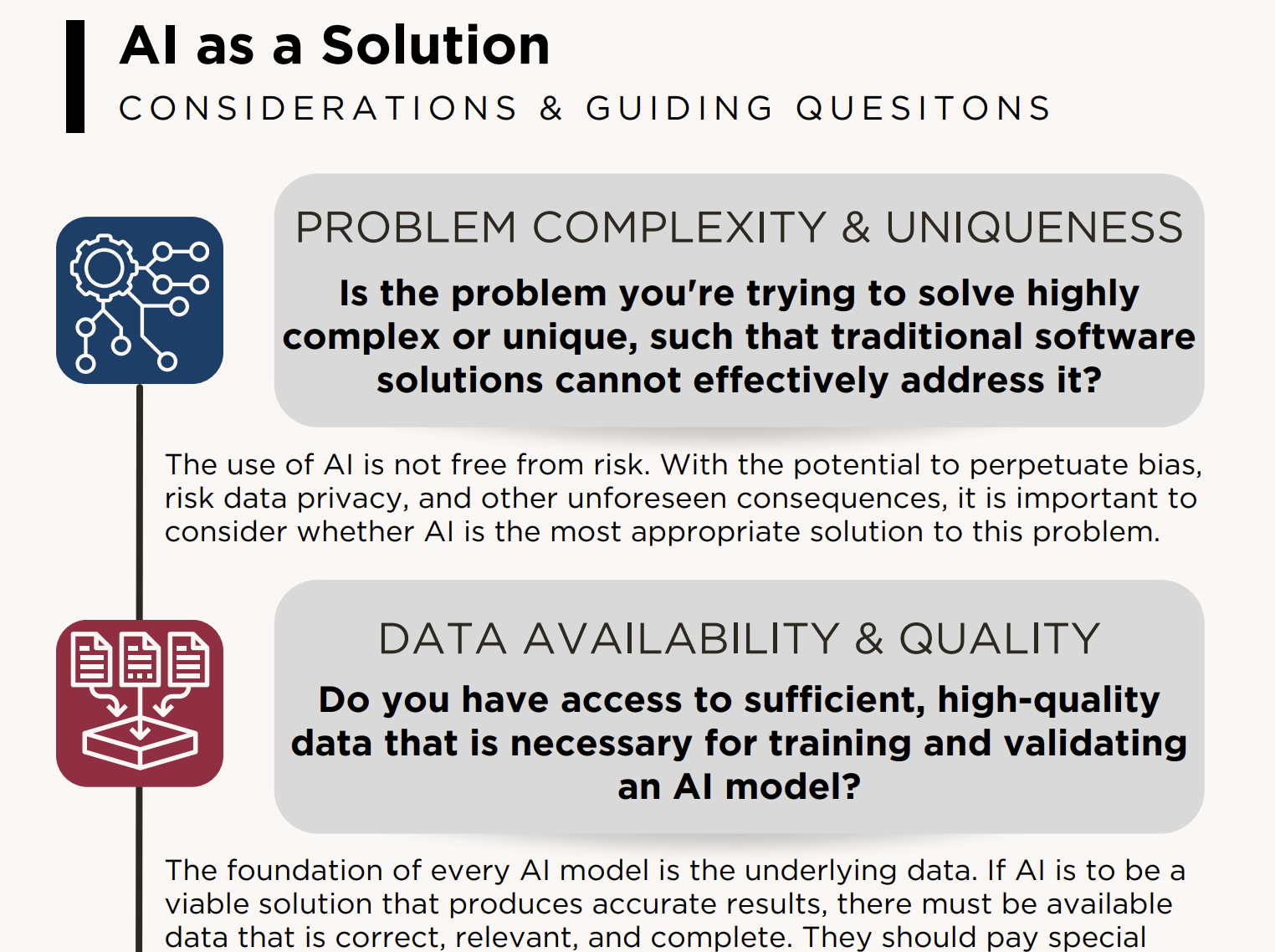
This guide contains context and questions to consider when assessing whether AI is the best solution for your defined problem.
These considerations include:
- Problem complexity and uniqueness
- Data availability and quality
- Computational resources
- Implementation needs
- Integration with existing systems
- Risk and bias introduction6
Exploratory Example
In this example, an institution considers AI as a potential solution to campus security challenges.
Problem Statement: Despite the importance of campus security, traditional access control measures, including security cards and monitoring, at campus entrances often face human capital challenges in effectively hiring, training and managing authorized personnel, leading to potential security breaches, unauthorized access, and safety concerns. In light of these issues, there is a need for a more robust and efficient security solution that can accurately identify individuals and enhance overall campus safety.
Goals for the solution: 1) Improve Campus Security: Implement AI facial recognition technology to strengthen security measures and mitigate potential threats at campus entrances; 2) Enhance Access Control: Develop a system that accurately identifies authorized personnel, students, and visitors while preventing unauthorized access; 3) Streamline Operations: Implement an efficient and user-friendly system that minimizes delays and congestion at campus entrances, improving the overall flow of pedestrian traffic.
Use Case: AI-powered facial recognition technology, with input images collected from student and staff ID images, will be used to limit access to campus buildings for authorized staff and students during specified time frames. The system will store access data, including access time, length of stay, frequency, and more, to enhance campus security and inform decision-making.
Assessment of AI as a Solution: In this scenario, the institution should consider cost-effectiveness and ease of implementation with the availability of alternatives. Access mechanisms such as RFID chips on student or staff ID cards may be a more appropriate solution than implementing facial recognition technology. While facial recognition offers advanced security features, its implementation often involves significant costs, complex infrastructure, and potential privacy concerns. In contrast, RFID technology provides a simpler and more affordable solution that can be quickly rolled out with minimal disruption to existing systems, making it the preferred choice for many organizations seeking to enhance campus security without the complexities associated with AI-based solutions.
In considering these options, the institution should weigh several key equity considerations. Based on a recent analysis of facial recognition technology, this AI solution may be less accurate for Black students and staff members, limiting access to campus buildings at essential times, hindering their educational efforts, and making the campus environment less inclusive, impacting their sense of belonging. Additionally, there are significant data risks associated with the facial recognition data stored by the system, including privacy concerns and misuse of data inputs and AI outputs. Finally, because all students and staff need access to campus spaces, there would be no option to ‘opt-out’ of this AI solution, limiting the autonomy of users against current regulatory guidance for AI use.
- Santosh Kataram. (2023). Applying AI: How to define valuable AI use cases. Columbusglobal.com; Columbus Global. columbusglobal.com
- Magoulas, R. (2020). AI adoption in the enterprise 2020. O’Reilly Media. oreilly.com
- Setting goals and proving success in AI projects. (2022). Xomnia.com. xomnia.com
- Kästner, C. (2022). Setting and Measuring Goals for Machine Learning Projects. Medium; Medium. ckaestne.medium.com
- Andrea Di Stefano. (2021). AI use cases: 3 rules to find the right one for your business. Itransition.com. itransition.com
- 5 AI Implementation Strategy Building Tips.(2023).Turing Blog. turing.com
Overview: Purpose and Ideation
The purpose and ideation phase sets the direction and foundation for your AI solution, aligning it to the needs of those most impacted by its outputs. Considering equity throughout the ideating activities (defining the problem, setting goals, and aligning use cases) creates the conditions for an AI system that prioritizes fairness, inclusivity, and transparency.
As chief information officers increasingly experiment with AI projects, nearly half of these initiatives fail to successfully transition from pilot phase to full-scale implementation.1 One source of these failed deployments is the misalignment of business needs with the AI use cases, and research identified it as one of the most significant barriers to AI adoption.1 2 Defining clear, equity-aligned use cases begins with an in-depth exploration of the problem and how the objectives for the AI solution fit within the broader context of organizational goals.